viriya
International Captain
Introducing cricodds: the first step in my plan for a comprehensive hub for live match odds and detailed analysis.
The tool gives you match odds based on historical scenarios for limited over matches, with detail on where the numbers come from, and input options and graphs for better customization.
To illustrate with an example, say you're following an ODI match where India is chasing, 4 down and need 80 runs in 10 overs. Given those inputs, cricodds gives preliminary odds of 50% based on similar historical scenarios (given in the table).
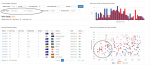
The column chart shows wins and losses by required run rate for similar match scenarios historically, and the bubble chart plots all historical scenarios over time by required run rate. Browsing the bubble chart tells you that chases such as these tended to end as losses more often in earlier ODIs (I've circled the relevant red dots).
To get better odds, you can customize the date range of historical matches to consider. Once you do that by setting the start date to 2005-08-10 (based on insight gleamed from the bubble chart - hover on the dots to get dates), the odds update to 54.44%:
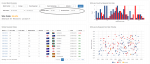
The next step to tweak the odds further is to input the teams involved. India is chasing vs Bangladesh, and since India's current team rating is significantly higher than Bangladesh, setting the teams as inputs increases the win odds even further to 62.69%:
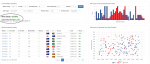
The team ratings adjustment is shown below the adjusted win odds as 8.25% so you can compare with the raw odds. Check this example here. The site now shows 63.19% for the win odds since it just picked up a few more recent similar ODIs that I just updated.
Currently it works for ODIs and T20s, with T20s using T20I and FT20 history for more data. The odds are calculated by using a simple ratio of the number of similar match scenarios that ended in wins and the total number of similar match scenarios.
Let me know what you guys think. These odds are intentionally somewhat simplistic, because a black box solution (say using machine learning methods) might give better odds, but will add a lot more complexity to the numbers.
Thoughts, ideas and criticisms welcome!
The tool gives you match odds based on historical scenarios for limited over matches, with detail on where the numbers come from, and input options and graphs for better customization.
To illustrate with an example, say you're following an ODI match where India is chasing, 4 down and need 80 runs in 10 overs. Given those inputs, cricodds gives preliminary odds of 50% based on similar historical scenarios (given in the table).
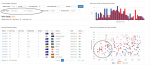
The column chart shows wins and losses by required run rate for similar match scenarios historically, and the bubble chart plots all historical scenarios over time by required run rate. Browsing the bubble chart tells you that chases such as these tended to end as losses more often in earlier ODIs (I've circled the relevant red dots).
To get better odds, you can customize the date range of historical matches to consider. Once you do that by setting the start date to 2005-08-10 (based on insight gleamed from the bubble chart - hover on the dots to get dates), the odds update to 54.44%:
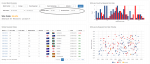
The next step to tweak the odds further is to input the teams involved. India is chasing vs Bangladesh, and since India's current team rating is significantly higher than Bangladesh, setting the teams as inputs increases the win odds even further to 62.69%:
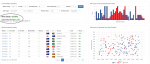
The team ratings adjustment is shown below the adjusted win odds as 8.25% so you can compare with the raw odds. Check this example here. The site now shows 63.19% for the win odds since it just picked up a few more recent similar ODIs that I just updated.
Currently it works for ODIs and T20s, with T20s using T20I and FT20 history for more data. The odds are calculated by using a simple ratio of the number of similar match scenarios that ended in wins and the total number of similar match scenarios.
Let me know what you guys think. These odds are intentionally somewhat simplistic, because a black box solution (say using machine learning methods) might give better odds, but will add a lot more complexity to the numbers.
Thoughts, ideas and criticisms welcome!